
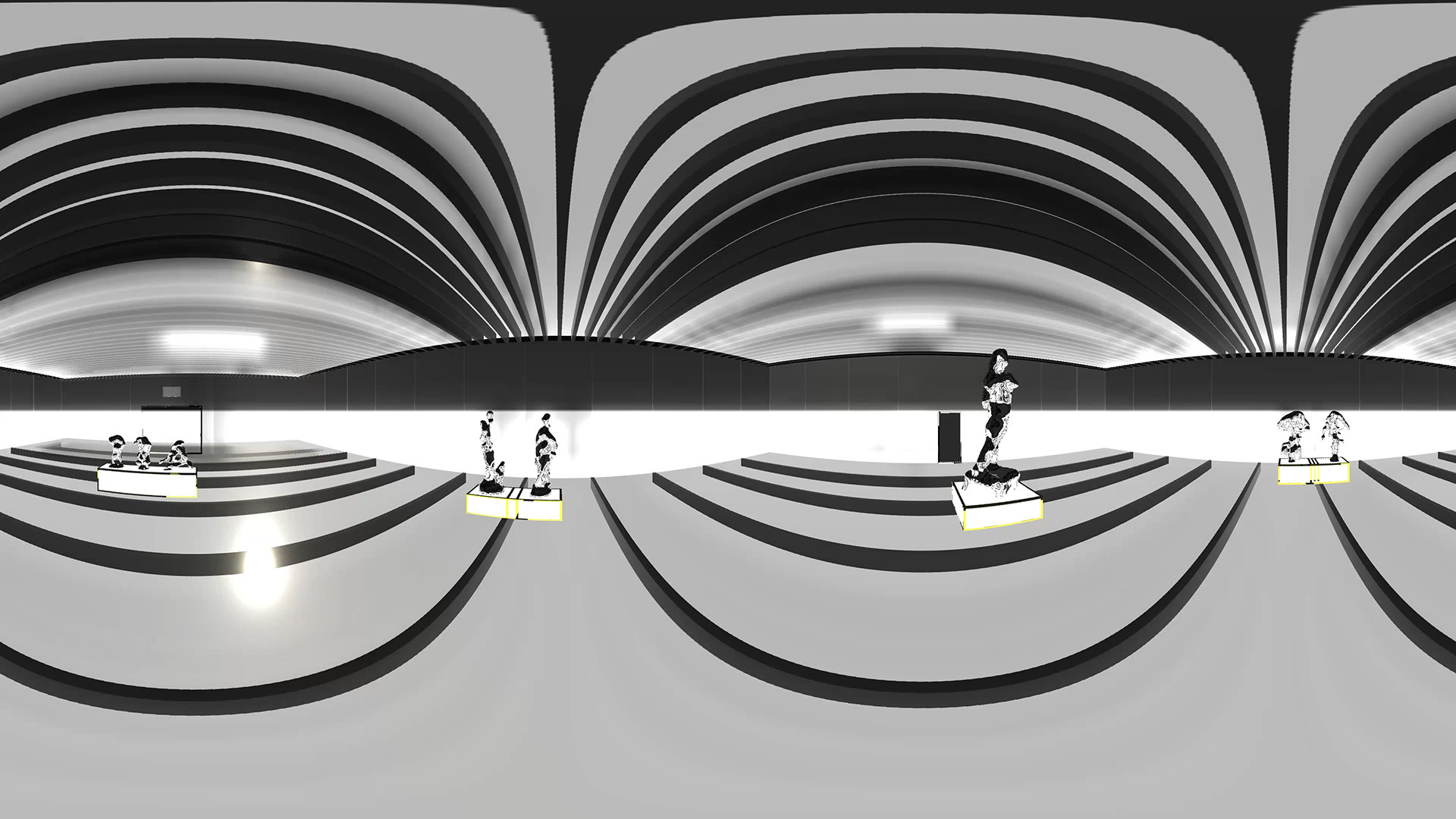
Mass spectrometry (MS) is the most widely used method for identifying or annotating metabolites in metabolomics. CFM-ID 4.0 is available at as a webservice and docker images can be downloaded at We demonstrate that this new version of CFM-ID (version 4.0) is significantly more accurate than previous CFM-ID versions, in terms of both EI-MS/MS spectral prediction and compound identification. This work improves CFM-ID’s ability to predict ESI-MS/MS spectra from compounds by: (1) learning parameters from features based on the molecular topology, (2) adding a new approach to ring cleavage that models such cleavage as a sequence of simple chemical bond dissociations and (3) expanding its hand-written rule-based predictor to cover more chemical classes, including acylcarnitines, acylcholines, flavonols, flavones, flavanones, and flavonoid glycosides. CFM-ID is one of the best-performing methods for compound-to-mass-spectrum prediction, and also one of the top tools for in silico mass-spectrum-to-compound identification.

To facilitate the generation of predicted spectral reference libraries we developed CFM-ID, a computer program that can accurately predict ESI-MS/MS spectrum for a given compound structure. These experimental libraries can be greatly extended by predicting the MS spectra of known chemical structures (which number in the millions) to create computational reference spectral libraries. As this typically involves matching a given MS spectrum against an experimentally acquired reference spectral library, this approach is limited by the coverage and size of such libraries (which typically number in the thousands). In the field of metabolomics, mass spectrometry (MS) is the method most commonly used for identifying and annotating metabolites.
